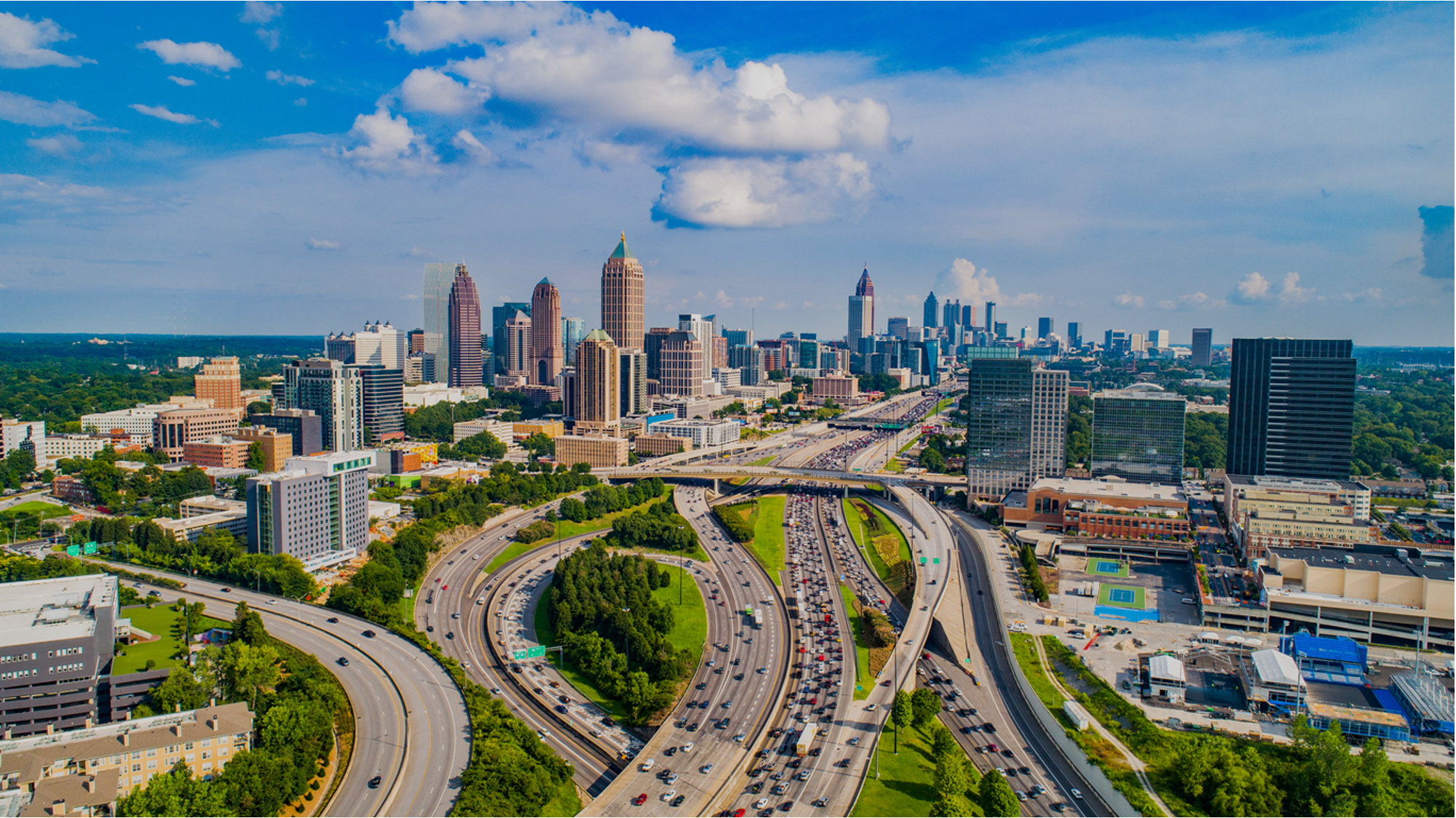
“Inference and Prediction for Safe Interaction” by Katherine Driggs-Campbell
Abstract: Autonomous systems, such as self-driving cars, are becoming tangible technologies that will soon impact the human experience. However, the desirable impacts of autonomy are only achievable if the underlying algorithms can handle the unique challenges humans present: People tend to defy expected behaviors and do not conform to many of the standard assumptions made in robotics. To design safe, trustworthy autonomy, we must transform how intelligent systems interact, influence, and predict human agents. In this talk, we’ll discuss how inferring hidden states (e.g., driver traits, pedestrian intent, occluded agents) coupled with robust prediction methods can be used to improve decision-making and control in interactive settings. These methods are used to generate safe interactions between vehicles and human road users (sometimes with guarantees), which are demonstrated on fully equipped test vehicles.
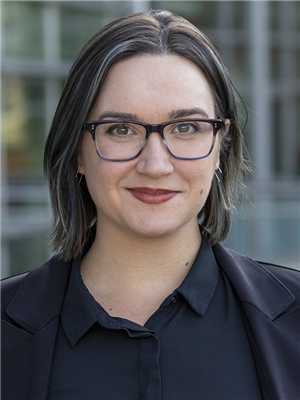
Bio: Katie Driggs-Campbell is currently an assistant professor at the University of Illinois at Urbana-Champaign in the Department of Electrical and Computer Engineering. Prior to that, she was a Postdoctoral Research Scholar at the Stanford Intelligent Systems Laboratory in the Aeronautics and Astronautics Department. She received a B.S.E. with honors from Arizona State University in 2012 and an M.S. from UC Berkeley in 2015. She earned her PhD in 2017 in Electrical Engineering and Computer Sciences from the University of California, Berkeley. Her lab works on human-centered autonomy, focusing on the integration of autonomy into human-dominated fields, merging ideas robotics, learning, human factors, and control.