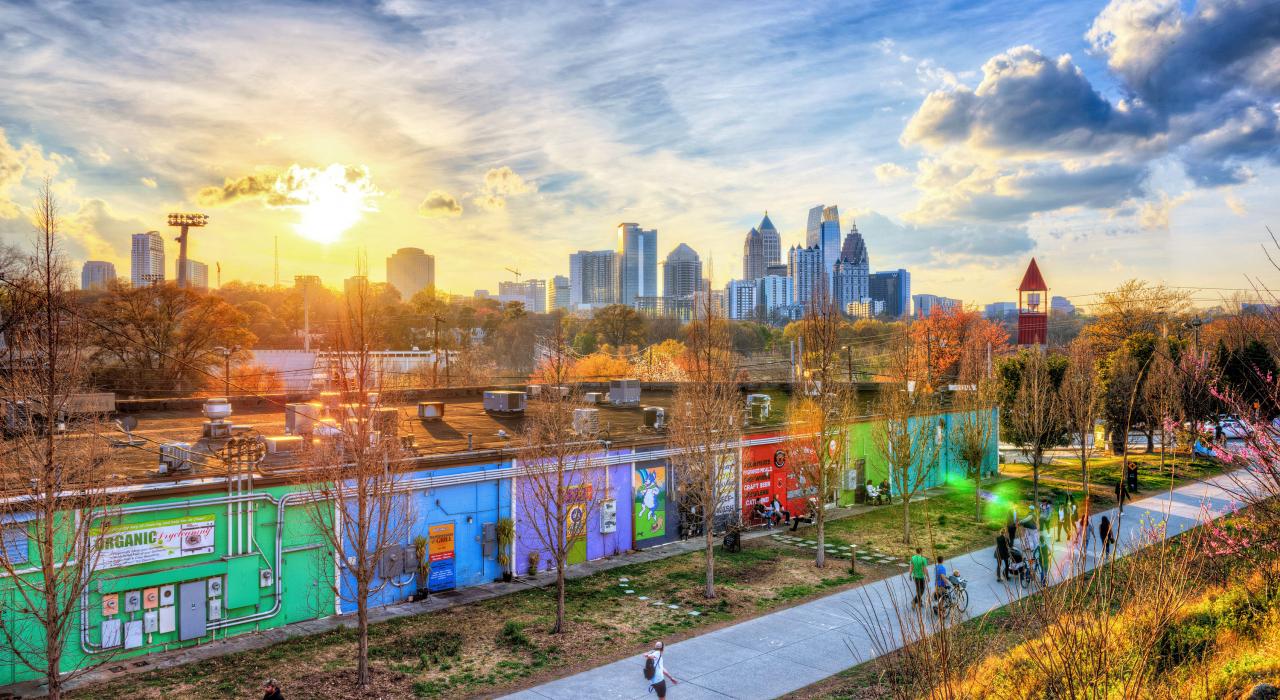
“Risk-aware Decision-making Modeling and Its Applications in Human-robot Interaction: A Regret Theory Approach” by Yue Wang
Abstract: Developing decision making models for robots in human-robot interaction (HRI) systems is fundamentally different from developing such models for fully autonomous robots. In HRI systems, robot developers no longer have the privilege to specify reward/cost functions following pure engineering principle of optimization. Instead, robots should use a model that can describe how humans make decisions for human-robot teams sharing the same decision model. In this talk, we present a descriptive model of human decision-making under risk that is psychologically grounded, neurobiologically evident, mathematically formal and behaviorally predictive, and to investigate the characteristics and capabilities of the model in HRI applications. We extend a non-expected utility theory, called regret/rejoice theory, by taking into account regret effects, probability weighting effects, and range effects. The goal is to enable robots to automatically make decisions under risk in a human-like way. To quantify the extended regret theory (RTx), we design a fuzzy logic controller to obtain desired data from individual decision makers. We further study the effects of risk awareness in a human-multirobot collaborative search task where multiple robots must be ordered to request human supervision. We cast the optimal ordering into multi-option choice problems and use RTx to make human-like risk-aware decisions. The results indicate that risk-awareness renders improved performance of robotic decision-making for HRI and RTx is a tractable embodiment of risk-awareness. Finally, we build a human-like lane-change decision model in highway traffic for better interaction between autonomous vehicles and the manual driven vehicles. The proposed computational model formulates the perception of the probabilities and outcomes (risk perception) and the driver risk propensity integrating Bayesian inference, Newtonian simulation, and RTx. The model was fitted and tested with empirical data from real traffic. The results support the idea that downplaying accident consequences may be the main contributor to risk-taking behaviors.
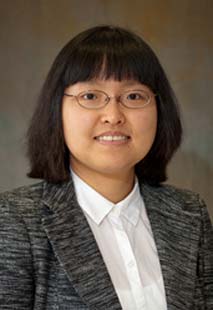
Bio: Dr. Yue “Sophie” Wang is the Warren H. Owen-Duke Energy Associate Professor of Engineering and the Director of the I2R laboratory at Clemson University. She received a Ph.D. degree in Mechanical Engineering from Worcester Polytechnic Institute in 2011 and held a postdoctoral position in Electrical Engineering at University of Notre Dame from 2011 to 2012. Her research interests include human-robot interaction systems, multi-robot systems, and cyber-physical systems. Dr. Wang has received the AFOSR YIP award, the NSF CAREER award, and the Air Force Summer Faculty Fellowship. Her research has been supported by NSF, AFOSR, AFRL, ARO, ARC, NASA, US Army, and industry. Dr. Wang is a senior member of IEEE, and member of ASME. She serves as the Associate Editor of the IEEE Robotics and Automation Magazine (RAM) and the ASME Journal of Autonomous Vehicles and Systems (JAVS). She is also a Technical Editor of the IEEE/ASME Transactions on Mechatronics (TMECH). Her work has been featured in NSF Science360, ASEE First Bell, State News, SC EPSCoR/IDeA Research Focus, and Clemson University News.