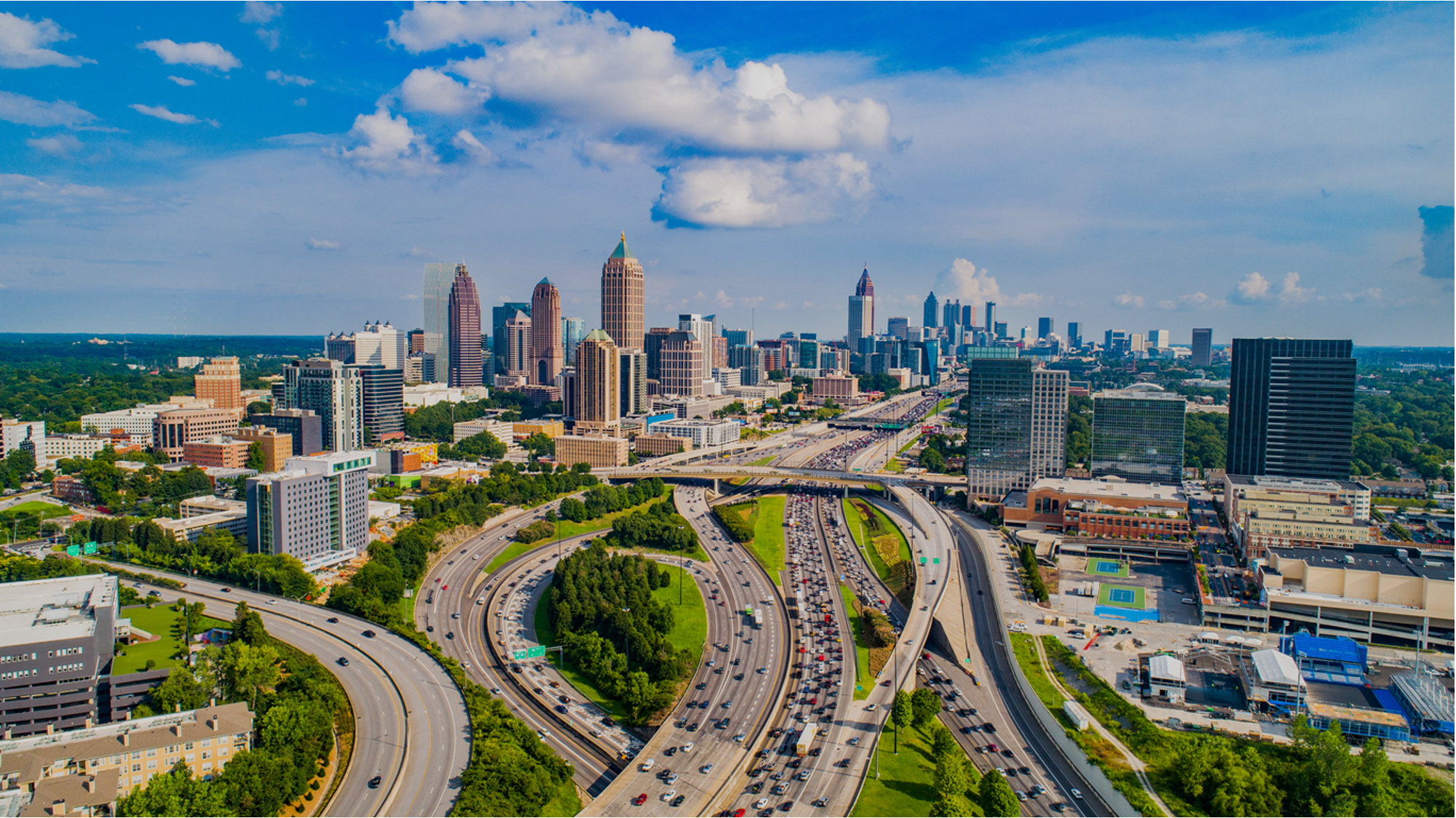
“Design and Evaluation for Interactive Autonomy in a Behavior-centric Closed Loop” by Wei Zhan
Abstract: Data-driven methods have been widely utilized for interactive behavior prediction and planning to enable autonomy. Typically, these learning-based models are designed and evaluated in an open-loop fashion, that is, to approximate the distribution of behavior datasets. However, an ego agent with the designed prediction and planning modules interacts with surrounding agents in a behavior-centric closed loop when actually utilized. The closed-loop safety, efficiency, comfort, social compatibility, etc. serve as the ultimate goals instead of distribution similarity to datasets. Therefore, the design and evaluation of learning-based behavior models should be conducted in a closed-loop fashion with reactive agents’ behavior and critical scenarios generated. In this talk, deep-generative-model-based methods are presented for generating human-like and critical reactive behavior and scenarios of surrounding agents. In addition, the closed-loop evaluation framework is constructed for learning-based behavior models based on densely interactive behavior datasets such as INTERACTION dataset we proposed. Results and analysis demonstrate performance difference and its essential reasons when state-of-the-art behavior models from prediction challenges based on our dataset are evaluated in open and closed loop. Finally, closed-loop design of learning behavior models is addressed based on framework and analysis of evaluation.
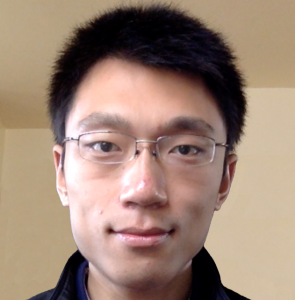
Bio: Wei Zhan received his Ph.D. from UC Berkeley in 2019. He is a Postdoctoral Scholar working on interactive prediction and planning, as well as understanding interactive scenes and behavior for autonomy with Professor Masayoshi Tomizuka at UC Berkeley. He published 55 papers on autonomy in flagship journals and conferences in various research communities including control, robotics, intelligent transportation system (ITS), machine learning and computer vision. One of his publications received Best Student Paper Award in IV 2018, a flagship conference of IEEE ITS Society. He served as an Associate Editor in IV 2019-2021. He also served as a lead organizer of a series of workshops on interactive prediction and planning as well as behavior data and evaluation in IROS 2019, IV 2019 and IV 2020. He is the leading author of the INTERACTION dataset with highly interactive behavior in various complex scenarios with international locations, and led a team organizing prediction challenges based on the dataset in NeurIPS 2020 and ICCV 2021.