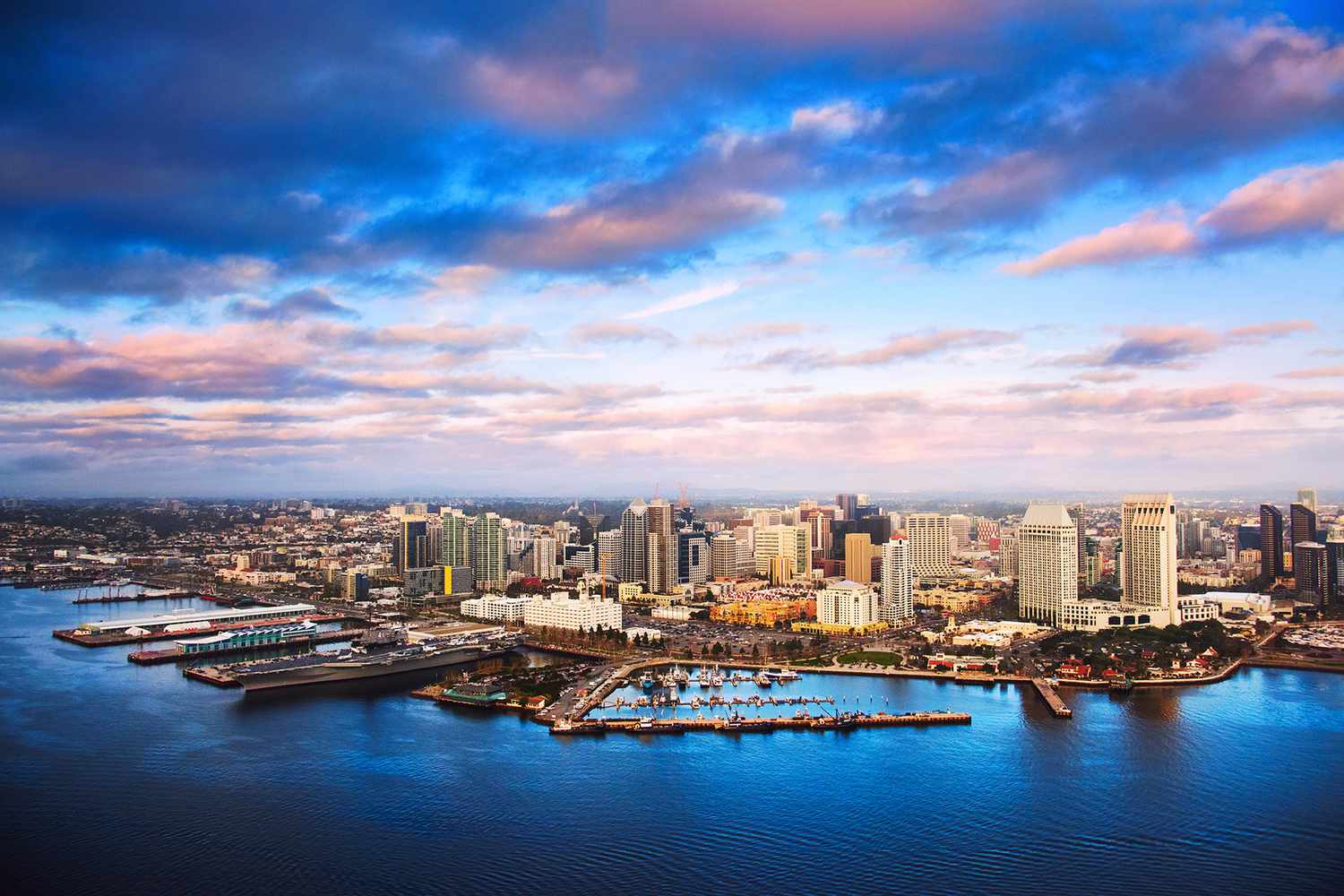
“Highway Driving Policy Learning Algorithms with the Awareness of the Driver and Neighbor Vehicles” by Fangjian Li
Abstract: Being aware of the presence of humans, autonomous driving policy learning algorithms can achieve a higher level of safety. In this talk, we focus on two vital human roles. First, as experts, experienced drivers can teach autonomous systems safe driving practices. To achieve this imitation learning task, we propose an innovative approach that combines inverse reinforcement learning (IRL) with safety awareness. Our method integrates control barrier function (CBF) analysis with a safety critic network to prioritize safety considerations. A safety regulator is introduced within the discriminator network to further discourage risk-seeking behaviors. Second, anticipating potential adversarial behavior from neighboring vehicles, we present a novel two-player-zero-sum Markov game (TZMG) based policy learning algorithm. This approach effectively handles corner cases in driving scenarios. Notably, the proposed adversarial learning framework has a synchronized training process for both subject safe driving policy and adversarial driving policies of neighbors, which significantly outperforms the vanilla simulation-based reinforcement learning approach.
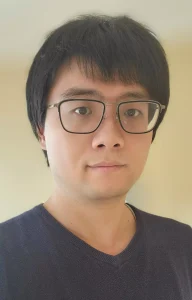
Bio: Dr. Fangjian Li received his Ph.D. degree in Mechanical Engineering Department at Clemson University (May 2022), and masterโs degree in Automotive Engineering at Clemson. His research focuses on the human-aware autonomous driving algorithms, i.e., how the presence of human drivers can affect or help the autonomous driving systems. The techniques involved in his research are (inverse) reinforcement learning, imitation learning, optimal control, and Bayesian inference. Dr. Li is working as a development engineer in Audi automated driving development office in San Jose.