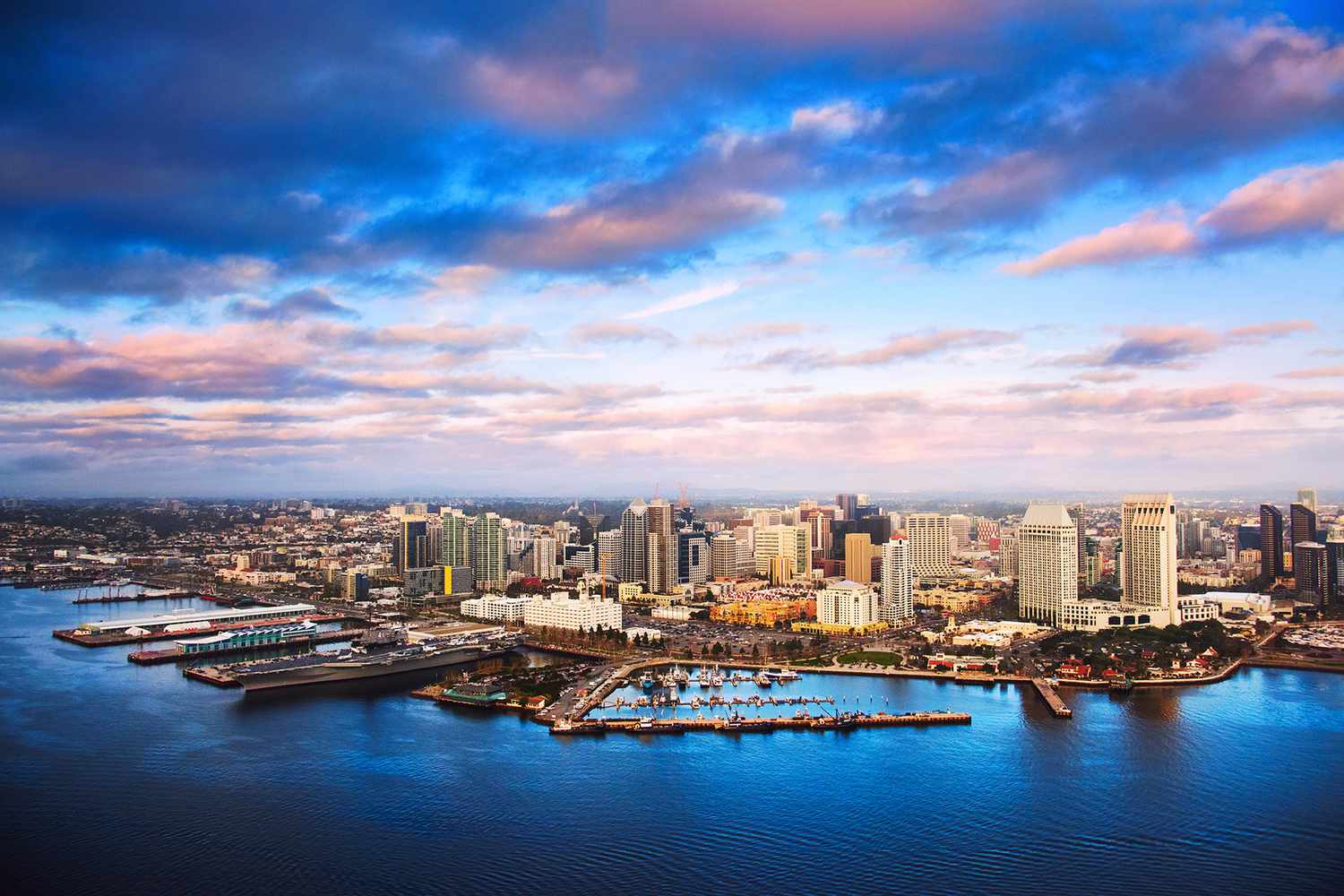
“Network-based Neurophysiological and Psychophysiological Metrics of Human Trust Dynamics When Teamed with Autonomy” by Zhaodan Kong
Abstract: In many future military and civilian settings, human operators will interact with autonomous systems primarily in a supervisory control mode, as those systems will have high levels of autonomy and not require direct human control. A key component to enabling high-performing human-autonomy teams is ensuring that the human operator has properly calibrated trust in the autonomous system. Significant progresses have been made recently demonstrating the utility of electroencephalogram (EEG), functional near-infrared spectroscopy (fNIRS), heartrate and heartrate variability, and skin conductance as measures to model trust. However, one major limitation of state-of-the-art methodologies is that: they are insufficient in discovering and investigating fundamental mechanisms underlying human trust and its dynamics. Therefore, the goal of our collaborative project is to combine the powers of wearable neurophysiological measures, e.g., EEG, fNIRs, and psychophysiological measures, e.g., electrocardiograph (ECG), respiration, electrodermal activity (EDA), using machine learning techniques, for the development of trust metrics suitable for dynamic assessment. In this talk, we will present some preliminary results we have obtained toward this goal, specifically to assess the following three hypotheses with increasing complexity: (i) A properly selected set of local features of EEG measurements or fNIRS measurements, predicts human trust with a higher than chance accuracy; (ii) A properly selected set of global, networked-based metrics derived from EEG measurements predicts human trust with a higher accuracy than that of local features; (iii) A properly selected set of metrics derived from multiple measures of physiological and observable measurements (i.e., multi-modal) predicts human trust with a higher accuracy that that of a set of metrics derived from a single measure alone (i.e., uni-modal).
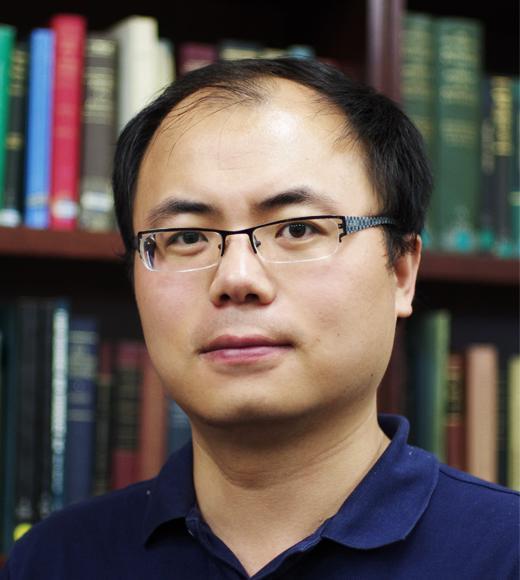
Bio: Zhaodan Kong is an Associate Professor in Mechanical and Aerospace Engineering at University of California, Davis. He is also affiliated with the Center for Spaceflight Research and the Center for Neuroengineering and Medicine at UC Davis. He received his Bachelor’s and Master’s degrees in Astronautics and Mechanics from Harbin Institute of Technology, Harbin, China, in 2004 and 2006, respectively, and his Ph.D. degree in Aerospace Engineering with a minor in Cognitive Science from the University of Minnesota, Minneapolis, MN, USA, in 2011. Before joining UC Davis in 2015, he was a Postdoctoral researcher at Boston University. His research focuses on control theory, machine learning, formal methods, and their applications to human-machine systems, cyber-physical systems, and neural engineering.