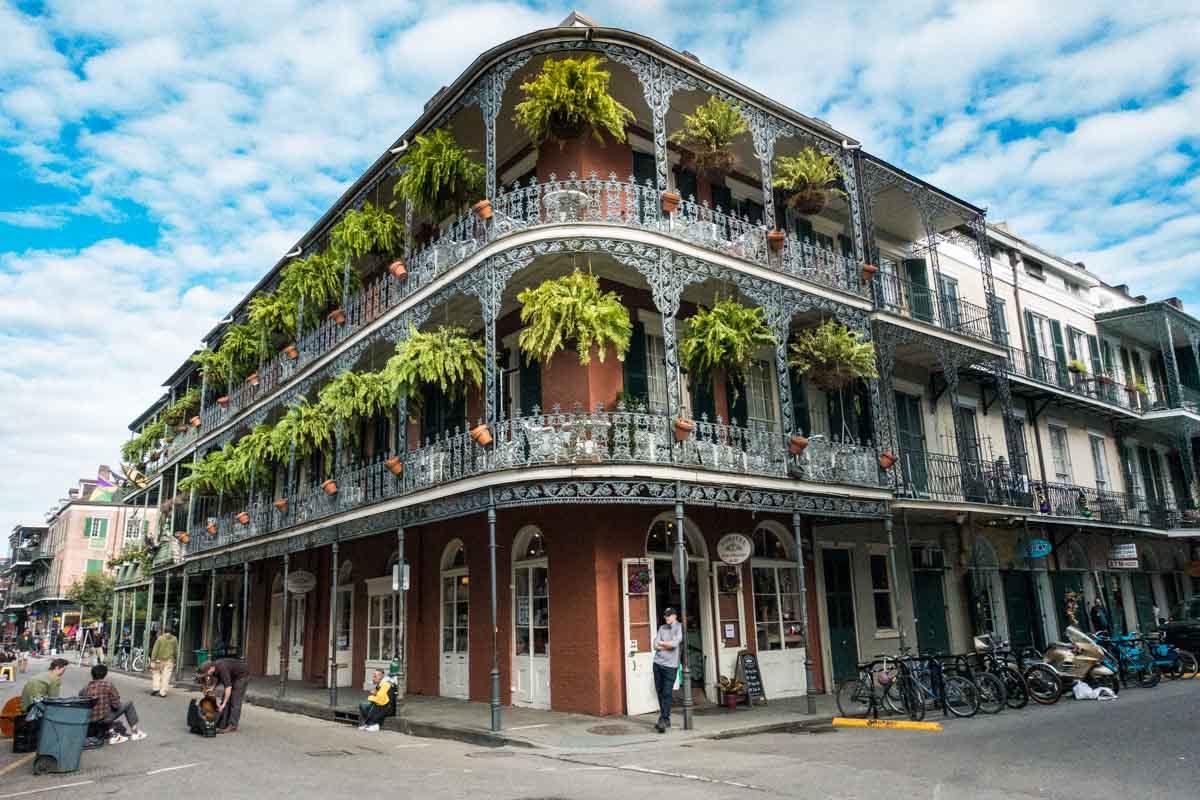
“Objective Learning for Human-Autonomy Teaming”
by Dr. Shaoshuai Mou
Abstract: Robots’ autonomy driven under optimal control heavily depends on the objective function to optimize corresponding to different missions. One efficient way for robots to acquire new skills is by learning such objective functions from demonstrations. When such demonstrations are from human experts, they are usually sparse in terms of only a small number of data points available, and inaccurate in the sense that only directional guidance is available. In this talk, we will first investigate methods based on inverse optimal control to enable robots to learn objective functions from sparse inputs. We then talk about techniques on how to integrate human inputs to correct robots’ performance, where such inputs will only contain directional corrections and will be given iteratively based on the robot’s performance. Simulations will also be provided to validate the proposed methods on motions of a robotic arm and path planning of quadrotors.
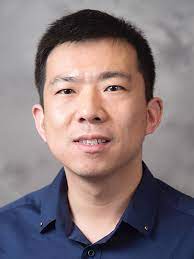
Bio: Dr. Shaoshuai Mou is an assistant professor at Purdue University. He received his Ph.D. in Electrical Engineering at Yale University in 2014 and then worked as a postdoc researcher at MIT till 2015. His research is in the area of control, optimization and networks with particular interests in multi-agent systems, distributed algorithms for control, optimization and learning, resilient autonomy, and human-robot autonomy.